Recommendation Tips About What Is Forecasting In Time Series Chart With 2 Y Axis
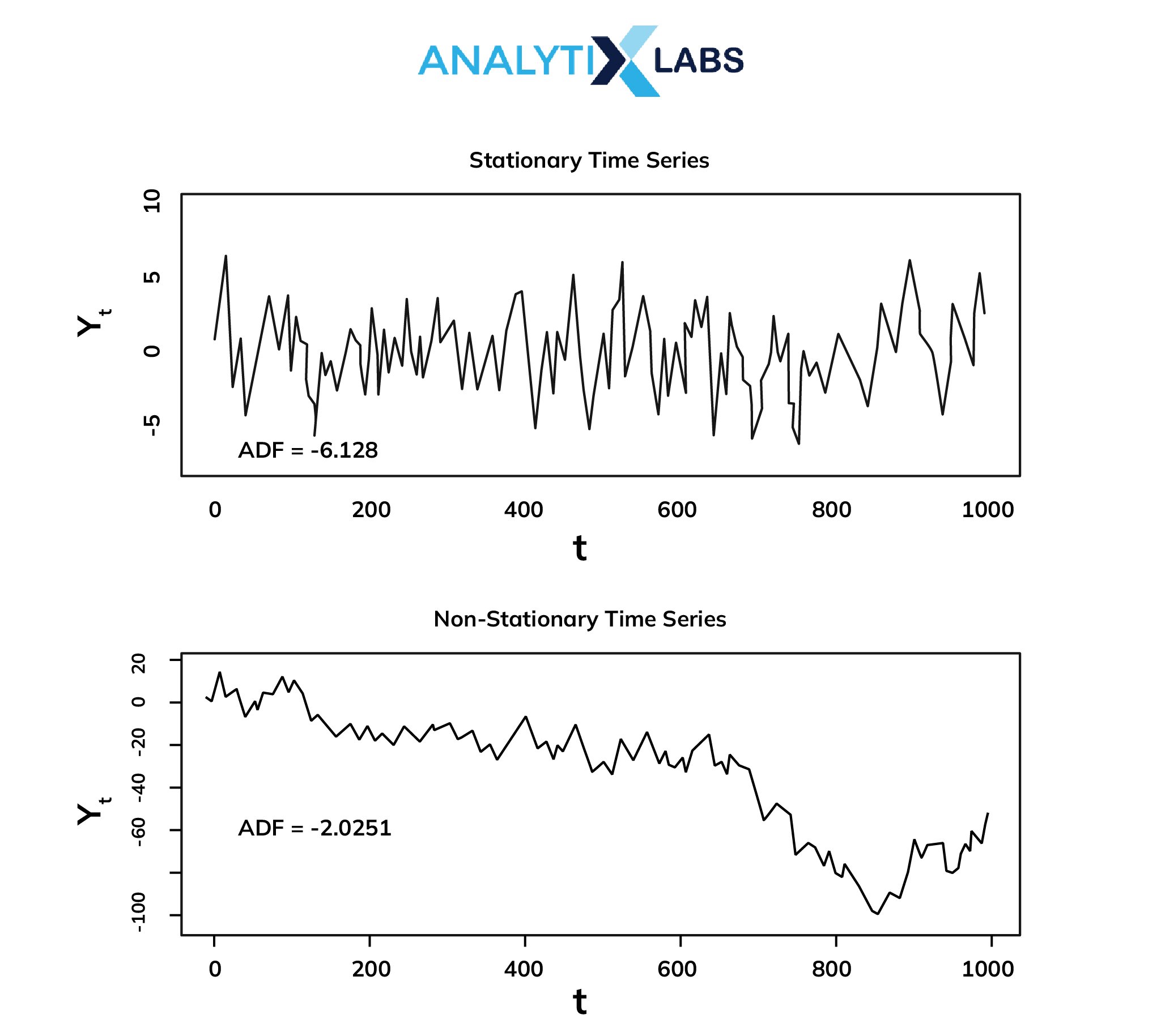
It can show likely changes in the data, like seasonality or cyclic behavior, which provides a better understanding of data variables and helps forecast better.
What is forecasting in time series. A wide array of methods are available for time series forecasting. Time series forecasting is a data science task that is critical to a variety of activities within any business organisation. We also find that despite their significant computational cost.
It helps businesses make informed decisions, optimize resources, and mitigate risks by anticipating market demand, sales fluctuations, stock prices, and more. Time series forecasting is a method of predicting future events by analyzing historical data. Time series analysis and forecasting are crucial for predicting future trends, behaviors, and behaviours based on historical data.
In this post, you will discover time series forecasting. Time series forecasting involves analyzing data that evolves over some period of time and then utilizing statistical models to make predictions about future patterns and trends. Standard definitions of time series, time series analysis, and time series forecasting.
This gives the impression that deep learning will solve all our problems for time series forecasting. The important components to consider in time series data. The three types of forecasting.
Forecast future values: In 2023 the company lost $4.45. What is time series forecasting?
Then in 2021, earnings grew to $6.06/share and grew again in 2022 to $8.34/share. Time series forecasting is a useful tool that can help to understand how. T he nation’s most advanced fleet of weather satellites is about to get an update.
However, there are other aspects that come into play when dealing with time series. Deep learning for time series forecasting receives a lot of attention. In investing, a time series tracks the movement of the chosen data points, such as a security’s price, over a specified period of time with data points recorded at regular intervals.
By analyzing data that we stored in the past, we can make informed decisions that can guide our business strategy and help us understand future trends. Remember to normalize your data if necessary, as lstm networks are sensitive to the scale of the input data. In a time series, time is often the independent variable, and the goal is usually to make a forecast for the future.
Time series forecasting is a technique for predicting future events by analyzing past trends, based on the assumption that future trends will hold similar to historical trends. Time series forecasting refers to the practice of examining data that changes over time, then using a statistical model to predict future patterns and trends. Many articles and scientific papers write about the latest deep learning model and how it is much better than any ml or statistical model.
Time series forecasting involves the collection of historical data, preparing it for algorithms to consume, and then predicting the future values based. Time series forecasting means to forecast or to predict the future value over a period of time. We’re trying to predict perhaps stock prices over time, asset prices, different macroeconomic factors that will have a large effect on our business objectives.