Simple Tips About How To Identify Trend And Seasonality In Time Series Data Add Secondary Axis Excel 2010
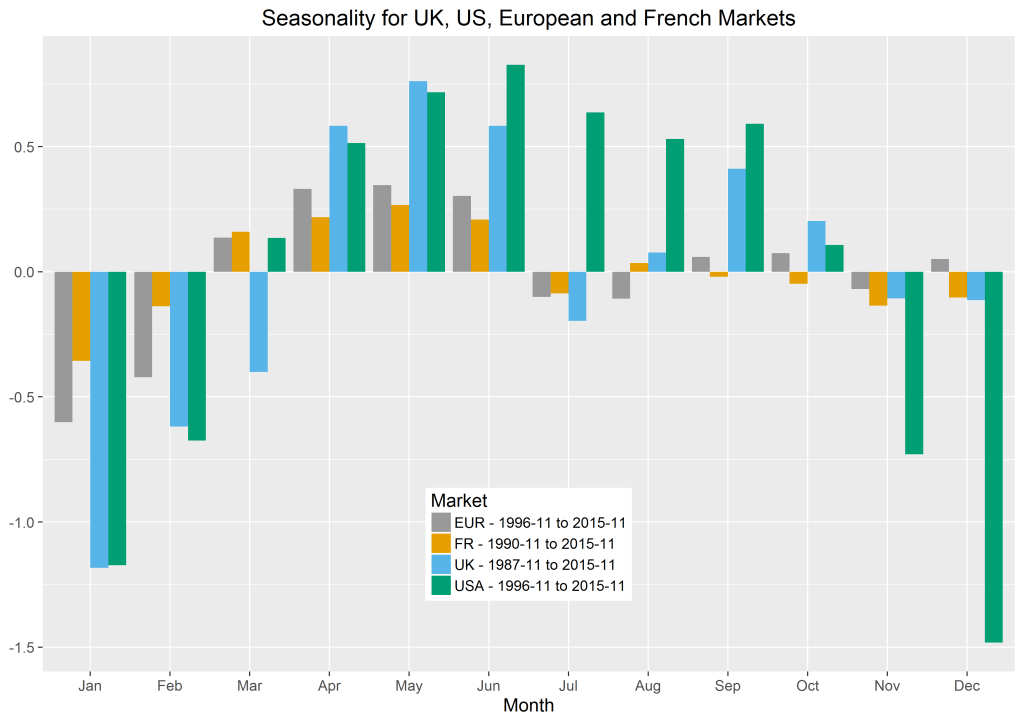
The definition of seasonality in time series and the opportunity it provides for.
How to identify trend and seasonality in time series data. How to identify trends & seasonality in time series data in r. Notice how time—depicted at the bottom of the below chart—is the axis. The first article of this series was about exploring both visual and statistical methods to.
Jan 2018 · 18 min read. As seen above, wiki gives very straight forward definition, any data in a sequential time order. The structure of decomposing time series into level, trend, seasonality, and noise.
As a part of a statistical analysis engine, i need to figure out a way to identify the presence or absence of trends and seasonality patterns in a given set of time series data. A moving window analysis involves calculating statistical properties over a sliding window of fixed length. In this article, we will embark on a journey to.
A really good way to find periodicity in any regular series of data is to inspect its power spectrum after removing any overall trend. Now lets take a look at the definition from investopedia. (this lends itself well to automated screening when the total power is normalized to a standard value, such as unity.)
The importance and types of trends that may exist in time series and how to identify them. How to use a simple differencing method to remove a trend. Have you ever looked at a time series and wonder if there are any repetitive patterns, outliers, or change points.
Suicide is a significant public health concern globally, with its varying rates influenced by numerous factors, including seasonal changes. Seasonality can come in different time intervals such as days, weeks or months. Seasonality detection allows analysts to recognize and understand recurring patterns within a time series which is valuable for interpreting historical trends and making informed predictions about future behavior.
Seasonality is one of the key components that make up a time series. In time series analysis, analysts record data points at consistent intervals over a set period of time rather than just recording the. How to detect seasonality, outliers, and changepoints in your time series.
After completing this tutorial, you will know: Time series data analysis is a powerful tool in understanding and forecasting trends in various domains, from finance to climate science. Now that we have a clear picture of the different models, let’s look at how we can break down our real estate time series into its trend, seasonality, and residual components.
A time series is a sequence of numerical data points in successive order. All it takes is kats and a few lines of code. Photo by insung yoon on unsplash.
Analyzing and dealing with seasonality is a key exercise in time series analysis. Last updated 12 months ago. In this article, we’ll describe three types of seasonality and how to detect them.